Everyone Wants Automation. Few Have Data Discipline
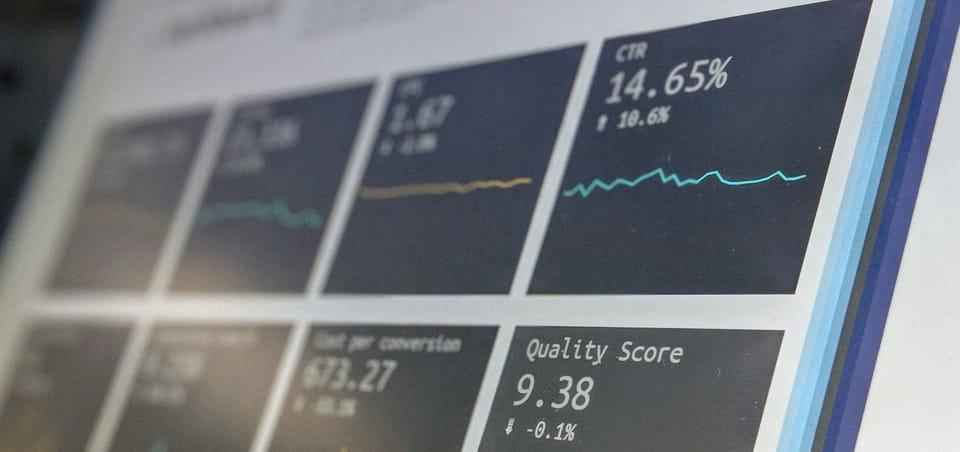
AI, automation, and business process tools are hotter than ever. Whether it's routing leads, streamlining approvals, or forecasting inventory, Ops leaders are under pressure to move faster, do more with less, and reduce errors across the board.
But here’s the catch: automation doesn’t work without clean, reliable data.
In fact, Gartner predicts that 74% of enterprises will embed AI into all operations by 20261 — but poor data quality is already the #1 reason automation initiatives fail to scale. Think of it like trying to build a high-speed rail system on crooked tracks. The tech may be sophisticated, but if your data is outdated, inconsistent, or scattered, it grinds everything to a halt.
Take it from real-world examples:
- A Fortune 500 company tried automating employee onboarding — and failed in 1 out of 5 cases due to inconsistent name formatting across HR and IT systems.
- A U.S. healthcare provider automated patient intake — but mismatched insurance codes caused thousands of coverage denials, requiring manual rechecks.
- Target couldn’t fully launch personalized recommendations until it unified browsing, purchase, and return data across platforms2.
If you're leading operations and thinking about automation, AI, or BPA — start with your data.
Why Data Is the Backbone of AI and BPA
1. AI Needs Data to Learn and Adapt
AI doesn’t “think” — it predicts. And what it predicts depends entirely on the quality of its training data. If you're using AI to forecast inventory, predict churn, or recommend actions, it’s only as smart as the data you feed it.
When that data is messy, incomplete, or biased, AI decisions go sideways. That means bad forecasts, false positives, or even compliance risks.
2. Automation Runs on Rules — and Data Drives the Rules
Business Process Automation (BPA) thrives on logic: “If this happens, then do that.” The triggers behind those workflows — form submissions, vendor names, contract values — are all data points.
If those inputs are inconsistent, automations break. For example, if your vendor is “Acme Corp” in one tool and “ACME Corporation” in another, you can’t automatically reconcile or approve payments without a human jump-in.
3. Data Gaps Destroy Trust
Nothing kills an automation rollout faster than mistrust in the system. If your team has to double-check results or fix errors caused by bad inputs, it defeats the whole point. Bad data = bad adoption.
What “Good” Data Looks Like for Operations
You don’t need a PhD in data science to build better data hygiene. Start by focusing on these key qualities:
- Consistent: Same formatting and naming conventions (e.g., dates, vendor names).
- Complete: No missing values in critical fields.
- Current: Updated regularly — stale data creates costly delays.
- Connected: Data flows between systems without duplication or silos.
- Contextual: Easy to interpret and understand for downstream logic or analysis.
🔍 Pro Tip: If you're using dropdowns and validation rules instead of freeform fields, you're already ahead of the game.
How to Build a Data-First Ops Culture
Here’s how high-performing Ops teams build trust in their data:
- Make data entry easy and foolproof. Use validation, default values, and standardized inputs.
- Align with RevOps, IT, and Product. Everyone must agree on what “source of truth” means.
- Train your team to treat data as operational infrastructure. It’s not just for reporting — it drives the work.
What Happens Without Good Data?
Let’s look at what poor data discipline costs:
Issue | Impact |
---|---|
Duplicate contacts or vendors | Wasted time, errors in payments |
Missing fields in CRM | Failed automations or poor follow-up |
Manual spreadsheet handoffs | Slows everything down, prone to human error |
Dirty data in AI models | Wrong recommendations, false alerts |
McKinsey found that companies lose 20–30% of potential revenue due to poor data quality3.
A Simple Data Readiness Checklist
Before rolling out automation or AI in your operations, ask:
✅ Do we have a clean and consistent source of truth?
✅ Are our data inputs standardized and validated?
✅ Can we trace each step in a workflow based on timestamps and statuses?
✅ Are we using the same definitions across departments?
If not — start here. Your automation will only be as successful as the data you build it on.
Wrapping Up: Good Data is an Ops Superpower
AI and automation can 10x your output — but only if your data is ready to support it. For Ops leaders, the path forward isn’t just about buying better software. It’s about building smarter foundations.
📌 Start with your data.
🛠️ Fix it at the source.
🚀 Then let automation scale your impact.
✅ Want to Audit Your Data Before Automating?
We’ve helped companies build clean data pipelines that fuel automation across every department. If you're considering automation but aren’t sure your data is ready, book a quick strategy call — we’ll show you how to get started.
📚 Sources
Footnotes
- Gartner, “AI Integration Across Enterprise Applications,” 2023. ↩
- Harvard Business Review, “How Target Rebuilt Its Data Infrastructure to Improve Personalization,” 2022. ↩
- McKinsey & Co., “The Data Opportunity in Digital Transformation,” 2021. ↩
- Gartner, “AI Integration Across Enterprise Applications,” 2023. ↩
- Harvard Business Review, “How Target Rebuilt Its Data Infrastructure to Improve Personalization,” 2022. ↩
- McKinsey & Co., “The Data Opportunity in Digital Transformation,” 2021. ↩